Introduction
Weather forecasting has always played a vital role in industries like agriculture, aviation, and disaster management. However, predicting weather accurately is challenging due to the complexity of meteorological data. AI-powered tools are transforming weather forecasting by processing vast amounts of data, identifying patterns, and generating reliable predictions in real-time.
This tutorial provides a comprehensive guide to developing AI tools for weather forecasting, adhering to SEO-friendly formatting to ensure higher visibility online.
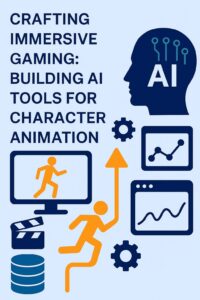
1. Why AI Tools Are Essential for Weather Forecasting
AI tools elevate weather forecasting by automating analysis and improving the precision of predictions.
Key Benefits of AI Weather Forecasting Tools
- Enhanced Accuracy: AI uses complex algorithms to deliver precise forecasts.
- Faster Predictions: Automates data processing, providing timely updates for critical decisions.
- Real-Time Insights: Incorporates live data to adapt predictions as conditions change.
- Scalable Solutions: Handles vast datasets from global weather stations and satellites.
- Disaster Mitigation: Identifies early warning signs for extreme weather events.
AI tools bridge the gap between traditional forecasting methods and modern technology-driven approaches.
2. Technologies Behind AI Weather Forecasting
Developing effective weather forecasting tools involves integrating cutting-edge technologies.
Core Technologies
- Machine Learning Models: Predict temperature, precipitation, and other metrics using historical data.
- Big Data Frameworks: Process enormous datasets from satellites, radar systems, and weather stations.
- Deep Learning: Analyzes patterns and trends for more accurate long-term predictions.
- IoT Integration: Connects sensors and devices for collecting real-time local data.
- Cloud Computing: Provides scalability for global weather monitoring systems.
These technologies work together to produce reliable, efficient, and scalable forecasting tools.
3. Preparing Data for AI Weather Tools
Data quality is crucial for accurate weather predictions. Proper organization and preparation ensure reliability.
Sources of Meteorological Data
- Satellite Data: Imagery and readings of cloud patterns, storm activities, and temperatures.
- Ground-Based Stations: Local weather data, such as humidity, wind speed, and rainfall.
- Historical Records: Long-term weather patterns for predictive modeling.
- IoT Sensors: Real-time updates on micro-climate data from devices in homes or farms.
- Climate Models: Data from simulations that predict large-scale environmental changes.
Steps to Prepare Data
- Clean the Data: Remove duplicates, incomplete entries, and errors.
- Standardize Formats: Ensure datasets align across sources for seamless integration.
- Label Features: Annotate data points like “temperature,” “pressure,” or “wind speed.”
- Integrate Multiple Sources: Combine satellite imagery, ground station data, and historical patterns.
Prepared data allows AI models to produce reliable and actionable forecasts.
4. Training AI Models for Weather Forecasting
The training phase is essential for creating tools that deliver accurate predictions based on complex datasets.
Best Practices for Model Training
- Supervised Learning: Use labeled weather data to teach the AI to predict specific metrics, like rainfall probabilities.
- Unsupervised Learning: Discover hidden trends and relationships in weather patterns.
- Reinforcement Learning: Improve forecasting accuracy by simulating various weather scenarios.
- Feedback Loops: Continuously refine predictions based on real-world outcomes.
With proper training, AI tools become highly adaptive and precise in their forecasts.
5. Deploying AI Weather Forecasting Tools
Deployment involves making your tool accessible to users while ensuring real-time functionality.
Strategies for Deployment
- Cloud Platforms: Offer global scalability and accessibility for meteorologists and industries.
- Mobile Integration: Provide apps with live updates for farmers, pilots, or disaster management teams.
- Interactive Dashboards: Visualize weather patterns and predictions in user-friendly formats.
- API Connectivity: Enable integration with other forecasting or climate monitoring tools.
- Real-Time Alerts: Deliver instant notifications for severe weather warnings.
Deployment should prioritize usability and scalability to maximize its impact.
6. SEO Optimization for AI Weather Tools
A strong SEO strategy ensures your tool reaches the right audience effectively.
SEO Best Practices
- Targeted Keywords: Use terms like “AI weather forecasting tools,” “real-time climate predictions,” and “smart meteorology solutions.”
- Content Creation: Publish blogs, tutorials, and case studies showcasing the tool’s capabilities.
- Mobile Optimization: Ensure websites and platforms perform seamlessly on all devices.
- Collaborations: Partner with meteorology agencies or industries to establish credibility.
- Metadata Structuring: Optimize page titles, tags, and descriptions for search engines.
SEO strategies ensure your tool gains visibility among relevant users and industries.
7. Monitoring and Refining AI Tools
Continuous improvement keeps your tool accurate and relevant in changing weather conditions.
Metrics to Track
- Prediction Accuracy: Compare forecasts with actual weather outcomes.
- Processing Speed: Ensure rapid analysis for real-time updates.
- User Feedback: Gather inputs to enhance usability and precision.
- System Errors: Address issues like incorrect or delayed predictions promptly.
Refinement ensures your tool stays ahead of industry demands.
Conclusion
AI tools for weather forecasting are revolutionizing how industries and individuals prepare for environmental changes. By combining advanced technologies, quality data preparation, and seamless deployment, these tools offer solutions for precise, real-time forecasts that benefit sectors like agriculture, transportation, and disaster management.
Start creating your AI weather forecasting solution today and contribute to a smarter, more prepared future.