Introduction
Understanding customer behavior is at the heart of successful business strategies. With the help of Artificial Intelligence (AI), companies can now predict customer actions, preferences, and buying habits with unmatched accuracy. These insights are invaluable for personalizing experiences, improving retention, and maximizing revenue.
This article provides a comprehensive guide to building AI-powered tools for predictive customer behavior analysis, adhering to SEO-friendly formatting to boost visibility and engagement.
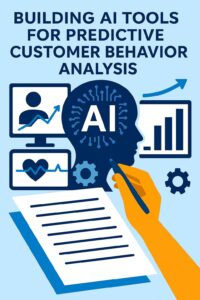
1. The Benefits of Predictive Customer Behavior Analysis
Predictive customer behavior tools give businesses a competitive edge by identifying trends and anticipating needs. Key advantages include:
- Personalized Customer Experiences: Tailor marketing campaigns, product recommendations, and interactions based on insights.
- Improved Customer Retention: Predict churn rates and implement proactive measures to retain customers.
- Optimized Inventory Management: Forecast product demand to avoid overstocking or understocking.
- Boosted Sales: Identify upselling and cross-selling opportunities with data-backed predictions.
- Enhanced Decision-Making: Leverage AI-driven insights to craft impactful business strategies.
These tools transform raw customer data into actionable business opportunities.
2. Core Technologies for Predictive AI Tools
To build effective predictive tools, businesses must utilize cutting-edge technologies tailored for customer behavior analysis.
Key Technologies
- Machine Learning Algorithms: Models such as Random Forest and Gradient Boosting predict future behavior based on historical data.
- Natural Language Processing (NLP): Analyzes text-based customer feedback and social media comments.
- Big Data Analytics Frameworks: Platforms like Apache Hadoop or Google BigQuery process massive datasets efficiently.
- Neural Networks: Deep learning models identify complex patterns in customer behavior.
- Predictive Analytics Platforms: Tools like IBM Watson or SAP Analytics Cloud deliver advanced forecasting capabilities.
These technologies empower AI tools to provide accurate, scalable, and actionable predictions.
3. Preparing Data for AI Models
The success of predictive behavior models depends on the quality and diversity of data used for training.
Data Sources to Consider
- Customer Purchase History: Analyze frequency, quantity, and type of purchases.
- Web Analytics: Examine user behavior on websites or apps, such as click-through rates and browsing time.
- Social Media Interactions: Insights from comments, likes, shares, and mentions.
- Feedback and Reviews: Text-based data revealing customer satisfaction or dissatisfaction.
- Demographic Information: Age, location, income levels, and preferences to segment users.
Steps for Data Preparation
- Clean and Normalize: Eliminate duplicates, errors, and inconsistencies in datasets.
- Feature Engineering: Extract actionable metrics, such as loyalty indicators or engagement rates.
- Diverse Data Integration: Combine data from multiple sources for comprehensive analysis.
- Privacy Compliance: Ensure that data collection and usage comply with regulations like GDPR.
Quality data sets the stage for reliable predictions and deeper insights into customer behavior.
4. Training AI Models for Accurate Predictions
The training phase is critical for developing models that generate accurate forecasts.
Training Best Practices
- Supervised Learning: Use labeled datasets to teach AI to predict customer actions based on past behaviors.
- Unsupervised Learning: Discover hidden customer behavior patterns through clustering and dimensionality reduction.
- Real-Time Data Analysis: Incorporate live customer interactions to refine predictive capabilities.
- Continuous Learning: Enable models to adapt to new trends and preferences dynamically.
- Bias Mitigation: Regularly audit training data and algorithms to prevent skewed predictions.
Well-trained AI models empower businesses to make data-driven decisions with confidence.
5. Deploying AI Tools for Customer Behavior Analysis
To achieve real-world impact, deploy AI tools with a focus on usability and scalability.
Deployment Strategies
- Cloud-Based Platforms: Leverage cloud computing for scalability and global accessibility.
- API Integration: Connect predictive tools with CRMs, ERPs, or e-commerce platforms seamlessly.
- Real-Time Dashboards: Provide decision-makers with visual insights into customer trends and forecasts.
- Mobile Accessibility: Ensure tools are optimized for smartphones and tablets.
Deployment ensures AI tools are accessible, actionable, and adaptable across business operations.
6. SEO Optimization for AI Customer Behavior Tools
Effective SEO strategies ensure your predictive tools reach the right audience.
SEO Best Practices
- Keyword Optimization: Use phrases like “AI customer behavior tools,” “predictive analytics for sales,” and “AI-powered forecasting platforms.”
- Content Marketing: Publish blogs, tutorials, and success stories showcasing tool capabilities.
- Mobile-Friendly Design: Ensure platforms perform well across all devices.
- Collaborations: Partner with industry leaders for credibility and backlinks.
- Metadata Structuring: Create compelling page titles and descriptions optimized for search engines.
SEO ensures your tool gains visibility and credibility in competitive markets.
7. Monitoring AI Performance & Enhancing Models
AI tools need continuous improvements to maintain accuracy and relevance.
Performance Metrics to Track
- Prediction Accuracy: Evaluate how well AI forecasts align with actual customer behavior.
- Processing Speed: Ensure predictions are delivered promptly for real-time decisions.
- User Engagement: Monitor how businesses and customers interact with AI-driven insights.
- Error Rate Analysis: Identify areas for refinement and improvement.
Regular updates ensure AI tools remain efficient, adaptable, and impactful.
Conclusion
AI-powered tools for predictive customer behavior analysis are revolutionizing how businesses understand and engage their audiences. With advanced technologies, quality data, and strategic deployment, companies can create solutions that transform insights into actions, driving growth and customer satisfaction.